Remote Magnetic Navigation at Clinical Scales for Micro and Nano Robots
[ Plenary Talk ]Bradley J. Nelson, Multi-Scale Robotics Lab, ETH Zurich, Zurich, Switzerland
09:05-09:55,Friday, August 23, 2024 Meeting Room B
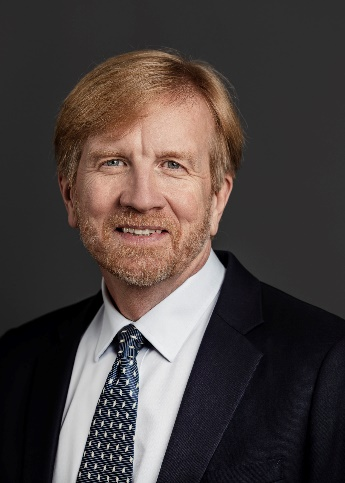
Abstract:
More than seventy years ago remote magnetic navigation (RMN) was proposed for guiding magnetically tipped catheters. The approach has been in clinical use for assisting surgeons during endocardial ablation procedures for more than two decades. We have recently developed a portable RMN system, more specifically an electromagnetic navigation system (eMNS), called the Navion that has been used for steering magnetically tipped catheters, guidewires, and endoscopes, as well as micro and nano robots. Our hope is that a clinically relevant system capable of guiding magnetic micro and nano robots will help propel the field of micro and nano robotics towards clinical adoption.
Biography:
Brad Nelson is the Professor of Robotics and Intelligent Systems at ETH Zürich and is the Chief Scientific Advisor of Science Robotics. He has over thirty years of experience in the field and has received several awards in robotics, nanotechnology, and biomedicine. He serves on the advisory boards several academic departments and research institutes across North America, Europe, and Asia. Prof. Nelson has been the Department Head of Mechanical and Process Engineering at ETH twice, the Chairman of the ETH Electron Microscopy Center, and a member of the Research Council of the Swiss National Science Foundation. He also serves on boards of three Swiss companies and is a member of the Swiss Academy of Engineering (SATW). Before moving to Europe, Nelson worked as an engineer at Honeywell and Motorola and served as a United States Peace Corps Volunteer in Botswana, Africa. He has also been a professor at the University of Minnesota and the University of Illinois at Chicago.
Vision-Based Tactile Sensing for Robot Manipulation and Learning
[ Plenary Talk ]
Michael Yu Wang, Great Bay University, China
09:55-10:45,Friday, August 23, 2024 Meeting Room B
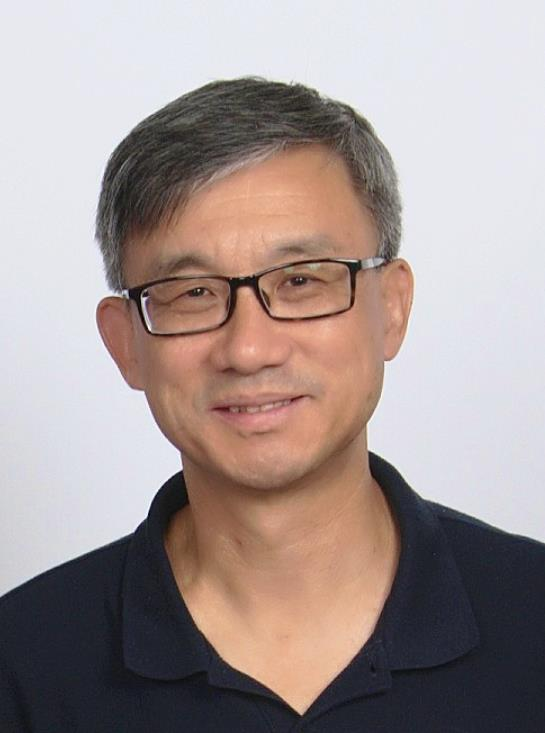
Abstract:
This presentation will give an overview of the trend in the development of embedded intelligence and human-centered robots. It will also focus on our research work on developing tactile sensors for robotic hands with dexterous and versatile capability for grasping and adaptive manipulation. Embodied intelligence requires and leverages a physical body, such as a robot, to achieve autonomous and rich physical interactions with the surrounding environment, which must be enabled by environment perception with vision and tactile sensing. In recent years we have seen rapid advancements in tactile sensor development and robot learning of agile grasping and manipulation with multi-modality information. We argue that the multi-modal manipulation capabilities of human-centered robots are essential for a feasible large-scale deployment of the future robotic technology with a potential of commercial success.
Biography:
Michael Yu Wang is a Chair Professor and the Founding Dean of the School of Engineering of the Great Bay University. He has served on the engineering faculty at University of Maryland, Chinese University of Hong Kong, National University of Singapore, Hong Kong University of Science and Technology, and Monash University. He has numerous professional honors–Kayamori Best Paper Award of 2001 IEEE International Conference on Robotics and Automation, the Compliant Mechanisms Award-Theory of ASME 31st Mechanisms and Robotics Conference in 2007, Research Excellence Award (2008) of CUHK, and ASME Design Automation Award (2013). He was the Editor-in-Chief of IEEE Trans. on Automation Science and Engineering, and served as an Associate Editor of IEEE Trans. on Robotics and Automation and ASME Journal of Manufacturing Science and Engineering. He is a Fellow of ASME, HKIE and IEEE. He received his Ph.D. degree from Carnegie Mellon University.
The Importance of Policy Representations
[ Keynote Talk ]Jens Kober, Associate Professor, TU Delft, Netherlands
14:00-14:35,Friday, August 23, 2024 Meeting Room B
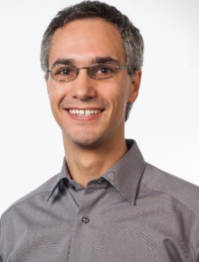
Abstract:
Learning motor skills in robotics has applications ranging from agriculture, manufacturing, to household environments and care. Imitation learning is one of the most prominent approaches in this field. Recent advances on large-scale models have enabled generalists, but are not yet suitable for highly specialized, dexterous, or personalized tasks. For those, we want to learn as efficiently as possible, on the one hand, i.e., from as few demonstrations as possible. On the other hand, it is paramount to ensure safety and stability of the learned movements and to enable cooperation with humans. Choosing the right representation of the policy is paramount to achieve these goals - a straightforward application of neural networks is typically neither data efficient nor ensures stability. In this talk I will explore several policy representations developed for efficient learning (also in settings with multiple reference frames), stability, and suitability for human-robot-interaction. All those ideas will be illustrated with real robot experiments.
Biography:
Jens Kober is an associate professor at the TU Delft, Netherlands. He worked as a postdoctoral scholar jointly at the CoR-Lab, Bielefeld University, Germany and at the Honda Research Institute Europe, Germany. He graduated in 2012 with a PhD Degree in Engineering from TU Darmstadt and the MPI for Intelligent Systems. For his research he received the annually awarded Georges Giralt PhD Award for the best PhD thesis in robotics in Europe, the 2018 IEEE RAS Early Academic Career Award, the 2022 RSS Early Career Award, and has received an ERC Starting grant. His research interests include motor skill learning, (deep) reinforcement learning, imitation learning, interactive learning, and machine learning for control.